Kadane’s Algorithm is a famous algorithm used for finding the maximum sum of a contiguous subarray within a one-dimensional numeric array. This problem is often referred to as the “Maximum Subarray Problem” and can be solved efficiently using Kadane’s Algorithm with a time complexity of O(n).
Table of Contents
Explanation
Here’s a step-by-step explanation of Kadane’s Algorithm:
- Initialization:
- Set two variables: max_so_far and max_ending_here.
- Both variables are initialized to the first element of the array.
- max_so_far keeps track of the maximum sum found so far.
- max_ending_here keeps track of the maximum sum of the subarray ending at the current position.
- Iterate through the array:
- Starting from the second element (index 1) to the end of the array, update max_ending_here to be the maximum of the current element itself or the current element added to max_ending_here.
- Update max_so_far to be the maximum of max_so_far and max_ending_here.
- Return the result:
- The value of max_so_far will be the maximum sum of the contiguous subarray.
Pseudocode for Kadane's Algorithm
function kadane(array):
max_so_far = array[0]
max_ending_here = array[0]
for i from 1 to length(array) - 1:
max_ending_here = max(array[i], max_ending_here + array[i])
max_so_far = max(max_so_far, max_ending_here)
return max_so_far
Example
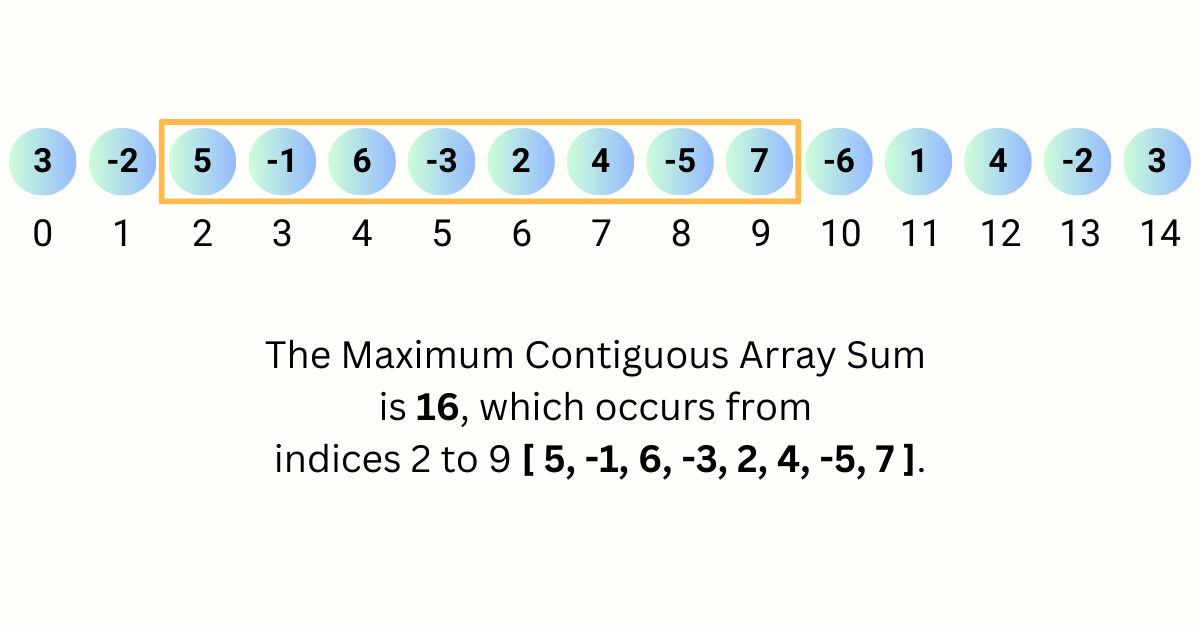
Let's consider an example array: [ 3, -2, 5, -1, 6, -3, 2, 4, -5, 7, -6, 1, 4, -2, 3 ].
- Initialization:
- max_so_far = 3
- max_ending_here = 3
- Iterate through the array:
- At index 1: max_ending_here = max(-2, 3 + (-2)) = 1 & max_so_far = max(3, 1) = 3
- At index 2: max_ending_here = max(5, 1 + 5) = 6 & max_so_far = max(3, 6) = 6
- At index 3: max_ending_here = max(-1, 6 + (-1)) = 5 & max_so_far = max(6, 5) = 6
- At index 4: max_ending_here = max(6, 5 + 6) = 11 & max_so_far = max(6, 11) = 11
- At index 5: max_ending_here = max(-3, 11 + (-3)) = 8 & max_so_far = max(11, 8) = 11
- At index 6: max_ending_here = max(2, 8 + 2) = 10 & max_so_far = max(11, 10) = 11
- At index 7: max_ending_here = max(4, 10 + 4) = 14 & max_so_far = max(11, 14) = 14
- At index 8: max_ending_here = max(-5, 14 + (-5)) = 9 & max_so_far = max(14, 9) = 14
- At index 9: max_ending_here = max(7, 9 + 7) = 16 & max_so_far = max(14, 16) = 16
- At index 10: max_ending_here = max(-6, 16 + (-6)) = 10 & max_so_far = max(16, 10) = 16
- At index 11: max_ending_here = max(1, 10 + 1) = 11 & max_so_far = max(16, 11) = 16
- At index 12: max_ending_here = max(4, 11 + 4) = 15 & max_so_far = max(16, 15) = 16
- At index 13: max_ending_here = max(-2, 15 + (-2)) = 13 & max_so_far = max(16, 13) = 16
- At index 14: max_ending_here = max(3, 13 + 3) = 16 & max_so_far = max(16, 16) = 16
- The maximum sum of the contiguous subarray is 16, which occurs from indices 2 to 9 [ 5, -1, 6, -3, 2, 4, -5, 7 ].
Python Code Implementation
def kadane(arr):
max_so_far = arr[0]
max_ending_here = arr[0]
for i in range(1, len(arr)):
max_ending_here = max(arr[i], max_ending_here + arr[i])
max_so_far = max(max_so_far, max_ending_here)
return max_so_far
# Example usage
temperature_change = [3, -2, 5, -1, 6, -3, 2, 4, -5, 7, -6, 1, 4, -2, 3]
print("Maximum contiguous sum is", kadane(temperature_change))
Advantages of Kadane's Algorithm
- Efficiency:
- Time Complexity: Kadane’s Algorithm operates in O(n) time, making it very efficient for large datasets.
- Space Complexity: It uses O(1) extra space, as it only requires a few additional variables.
- Simplicity: The algorithm is straightforward and easy to implement, involving simple iteration and comparison.
- Optimality: Kadane’s Algorithm guarantees finding the maximum sum of a contiguous subarray, providing an optimal solution for this specific problem.
Disadvantages of Kadane's Algorithm
- Limited Scope:
- It only solves the maximum subarray sum problem. For different types of subarray problems, modifications or entirely different algorithms might be needed.
- Handling Special Cases:
- In arrays where all elements are negative, Kadane’s Algorithm still works, but it might not be as intuitive.
- One-dimensional Focus:
- Kadane’s Algorithm is designed for one-dimensional arrays. For multi-dimensional arrays or other complex data structures, additional techniques or modifications are required.
Applications of Kadane's Algorithm
- Stock Market Analysis: To find the best time to buy and sell stocks for maximum profit, Kadane’s Algorithm can be applied to the array of daily price changes.
- Maximum Subarray Sum: Used in financial analysis, signal processing, and time series analysis to find the period with the maximum sum.
- Computer Vision: Used in algorithms for finding the largest sum rectangle in a matrix, which can be useful in image processing and computer vision tasks.
- Genomics: In bioinformatics, it can be used to find regions in DNA sequences that have the highest concentration of a certain property.
- Data Mining: For identifying patterns or trends in large datasets where the goal is to find contiguous segments with maximum sum or value.
Where Kadane's Algorithm Can Be Used
- Dynamic Programming Problems:
- Kadane’s Algorithm is a classic example of dynamic programming and can be used to introduce the concept.
- Optimization Problems:
- When dealing with optimization problems that require finding maximum sums or values in a linear structure, Kadane’s Algorithm is highly applicable.
- Real-time Systems:
- In systems that require real-time analysis of streaming data to identify peaks or maximum values quickly.
- Game Development:
- In game development, for algorithms that need to determine the best contiguous path or segment with the highest value (e.g., scoring maximum points in a segment).
Code Example for Applications
Here is a simple example demonstrating how Kadane’s Algorithm can be applied to the stock market analysis problem:
def max_profit(prices):
if not prices:
return 0
max_profit_so_far = 0
max_ending_here = 0
for i in range(1, len(prices)):
change = prices[i] - prices[i - 1]
max_ending_here = max(0, max_ending_here + change)
max_profit_so_far = max(max_profit_so_far, max_ending_here)
return max_profit_so_far
# Example usage
stock_prices = [100, 113, 110, 85, 105, 102, 86, 63, 81, 101, 94, 106, 101, 79, 94, 90, 97]
print("Maximum profit is", max_profit(stock_prices))
In this example, the max_profit function uses a variation of Kadane’s Algorithm to calculate the maximum profit that can be obtained from buying and selling a stock given daily price changes.